Guillaume Dalle
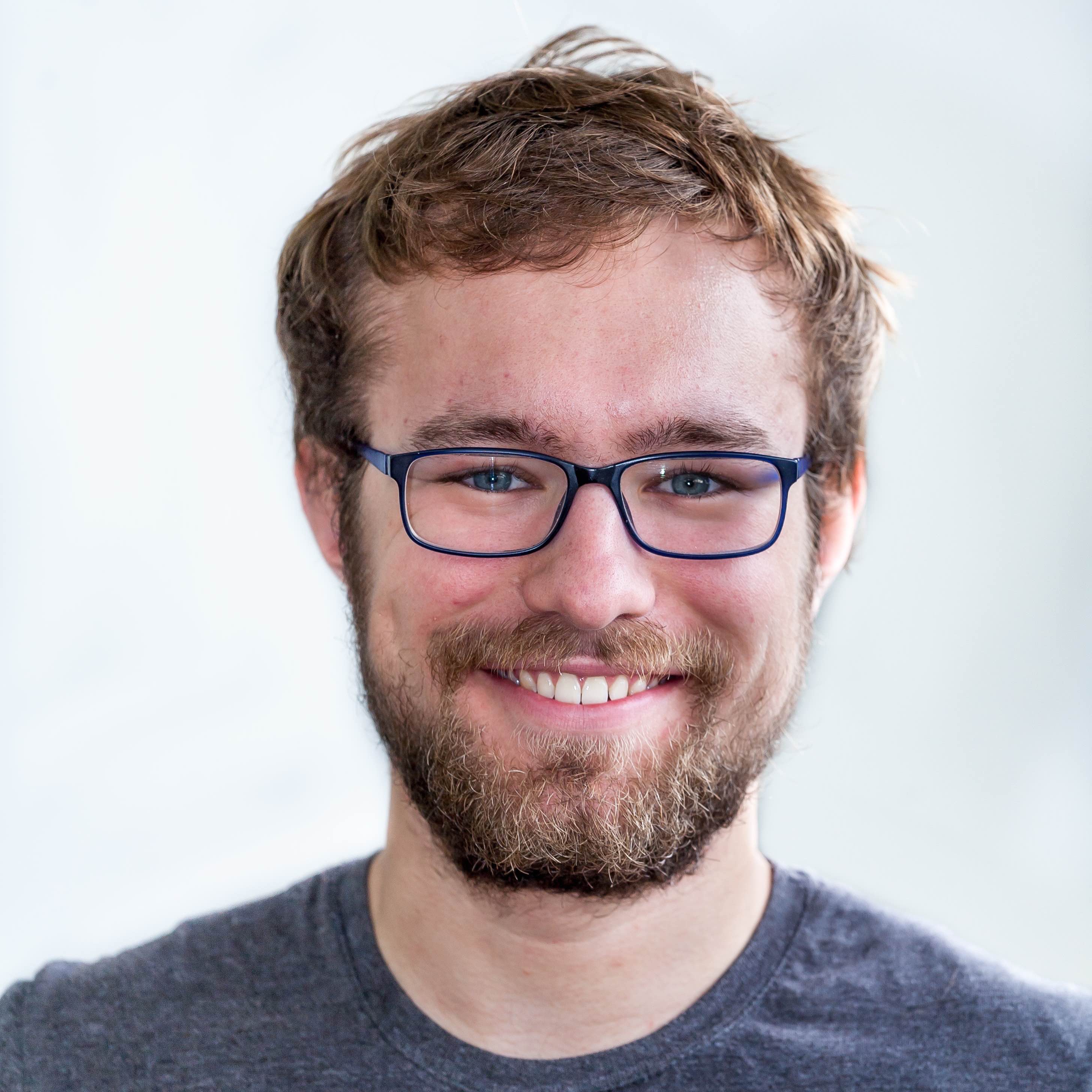
I am a postdoctoral researcher in machine learning at EPFL, working between the labs IdePHICS, SPOC and INDY. My current research focuses on the behavior of graph neural networks.
Between 2019 and 2022, I did my PhD at École des Ponts (CERMICS), followed by a visit to MIT (JuliaLab).
Check out my website PhD Resources for students and researchers